Jonathan Cavell
I am in a long-term fulfilling relationship with technology. Head-over-heels kind of love? Yes, you got it. I enjoy nothing more than discovering new technologies that enable businesses to achieve the previously unachievable. Unfortunately, too many companies view technology as just a set of systems required, completely separate from their core mission. I can’t entirely agree here. I believe, when technology is integrated appropriately, it makes everyone in the organization better at doing what they are good at.
That’s why I find it fulfilling to work with the best technologists in the world to make the best businesses in the world even better at what they do.
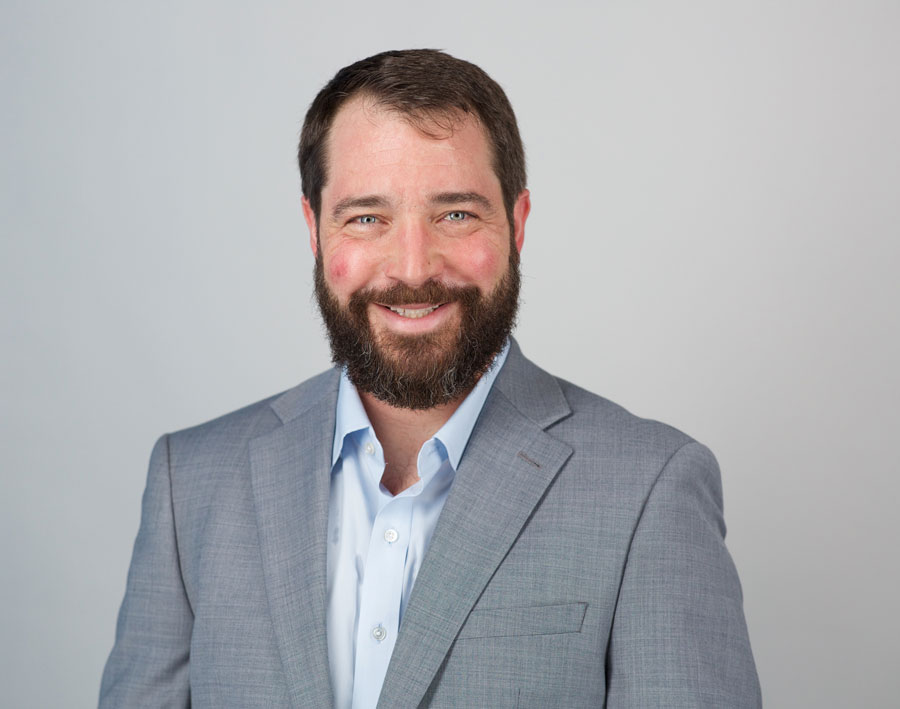
In spite of the fact that I am horrible at foreign languages, I have built a career as a translator. Let me explain. I don’t simply translate words from one language to another. I help people from entirely different planets speak to one another. I am capable of speaking to people from Planet Ops (I was an infrastructure architect at IBM and ran an Ops team at RBC), Planet Developer (I have worked as a Developer, Development Manager, and Agile Coach), and Planet Business (I have an MBA and a background as a product manager/product owner).
In a consulting capacity, I’ve helped customers turn their business problems into technical solutions. As a product manager, I’ve shown developers how to turn techie concepts into software. I have helped business people understand how a specific software could translate to the bottom line. As a development manager, I’ve used state-of-the-art DevOps, IaC, and Agile methods to develop unbelievably high-quality software predictably and efficiently.
I don’t have a lot of grey hairs (knock on wood), but I have worked hard to carefully develop the knowledge, reputation, attitude, and experience of a leader. I have brought this leadership to every job, role, and client I have helped in the last 20 years and am excited to manifest it to even more possibilities. I’m always looking for new opportunities to lead technologists and developers to assist them in pursuing what’s right for their business.